40% of the total IT budget will be spent on various types of AI testing applications in 2025, as IDC.com states. With their help, QA teams can automate up to 70% of routine tasks. Moreover, businesses benefit a lot from implementing “smart” tools in their IT workflows as they help to cut expenses by 50-70%.
What exact tools are businesses integrating into their QA processes? What is the future of AI in software testing? How do smart apps improve quality assurance, and what benefits do they bring? How does artificial intelligence transform QA expectations and processes? You will find the answers to all these questions in this article.
Traditional vs AI-based software testing: A quick comparison
Just 10-15 years ago, there were only classical approaches to testing, as there were no AI-powered tools that could enable improved process automation. Back in those days, the quality assurance process consisted mainly of automated tests with manually written scripts, as well as manual tests.
However, nowadays, AI-based applications are widely used and are gaining more and more popularity year after year. Let's take a closer look and compare the classic and AI-driven approaches to quality assurance, as well as their advantages and disadvantages.
Empower your AI to deliver unparalleled experience to your users
Traditional quality assurance: pros and cons
The classic approach to testing relies heavily on manual work by highly skilled QA engineers. This may include both manual testing and automated testing, where scripts are written by specialists using traditional programming languages like JavaScript. Various test automation frameworks, such as Selenium or similar tools, are often utilized. Traditional testing is typically a complex and time-consuming process, involving numerous phases, such as:
studying and analyzing requirements and specifications;
making a strategy;
developing test cases;
setting up the test environment;
running tests;
analyzing results, documenting, and finalizing processes.
Let's take a closer look at the advantages and disadvantages of the traditional approach.
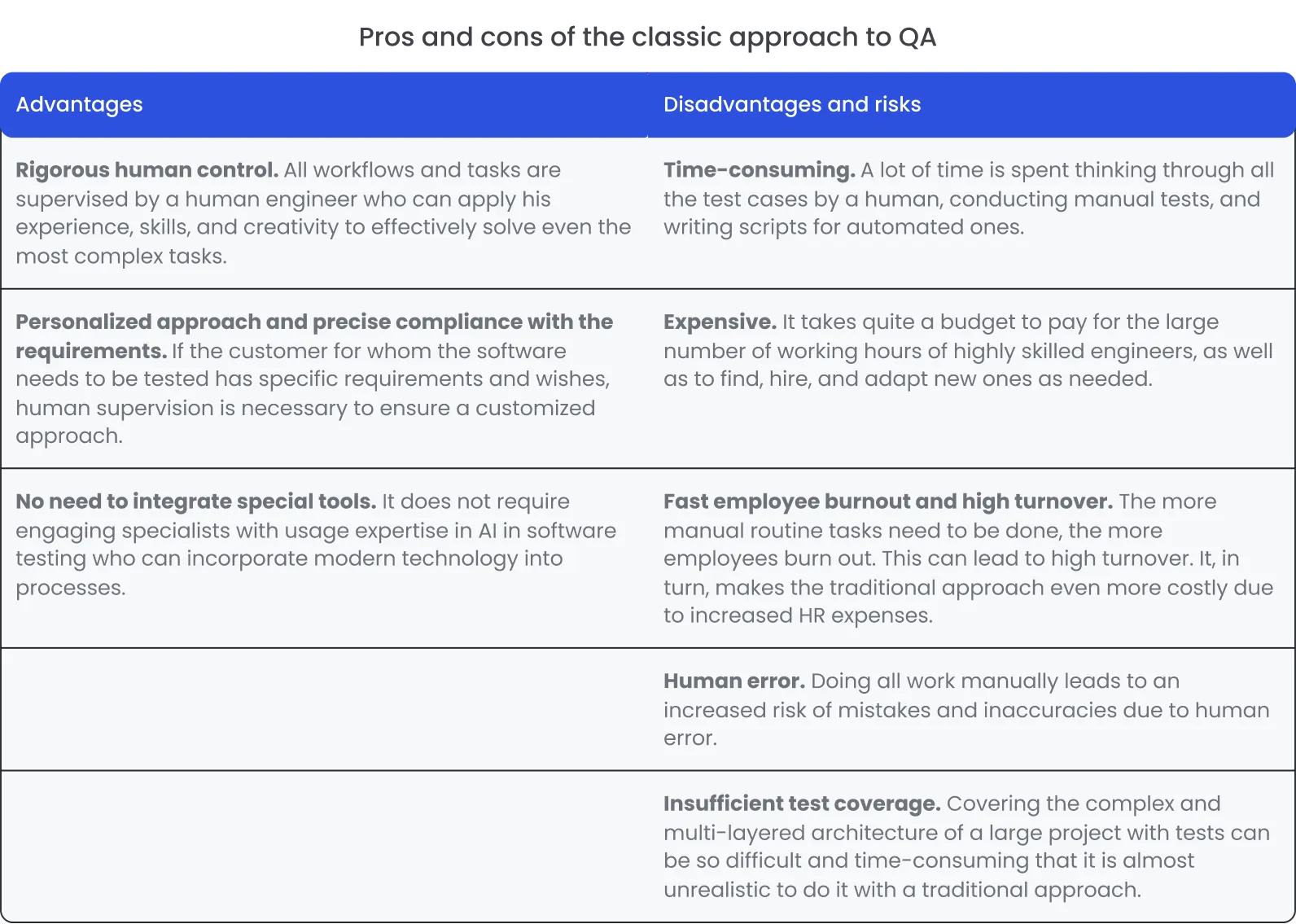
Benefits of AI-powered testing compared to traditional approach
With the help of AI, QA testing can become quicker, cheaper, and more accurate. Here are more details on the advantages of implementing smart tools:
Accelerating workflow. Automating tasks, for example, by prioritizing test cases or generating scripts for automated tests with AI, it is possible to significantly speed up the entire testing process. This gives a competitive advantage by reducing time-to-market.
Optimizing budget. AI-based tools can decrease the cost of building and maintaining a QA team. This becomes possible by reducing the working hours amount required for a complete testing cycle. It also helps reduce HR costs for finding and onboarding new employees due to a lower turnover rate.
Improving test coverage. AI helps find the code parts not covered with tests and fill these “gaps”. It is especially useful for products with a complex architecture.
Enhancing flexibility and scalability. A faster workflow gives an opportunity to gain more agility through more frequent updates and releases.
Mitigating some risks of a traditional approach. The classic approach involves such risks as human error or high levels of employee burnout and turnover. Implementing AI helps to minimize them.
Finding bugs earlier and more effectively. With the help of artificial intelligence, it is possible to find defects at earlier stages, for example, thanks to predictive algorithms, such as decision trees or linear regression. They can identify areas of code with the highest risk of bugs.
Human specialists + AI tools = high-quality testing
To achieve the most productive result possible, it is important to use both approaches together. AI tools can help speed up and improve processes. Meanwhile, human oversight can help make the work of “smart” assistants as accurate and efficient as possible, as well as solve manually some tasks that require special creativity and expertise.
The role of artificial intelligence in software testing in 2025
Artificial intelligence is gradually permeating all areas and workflows of IT: from development to customer support. And, of course, QA is no exception. AI-powered tools are very widely used in software testing. How and for what purpose do modern engineers use AI tools?
Existing ways of using AI in QA
According to the 2024 State of Testing Report, the most popular ways to use AI-driven tools are:
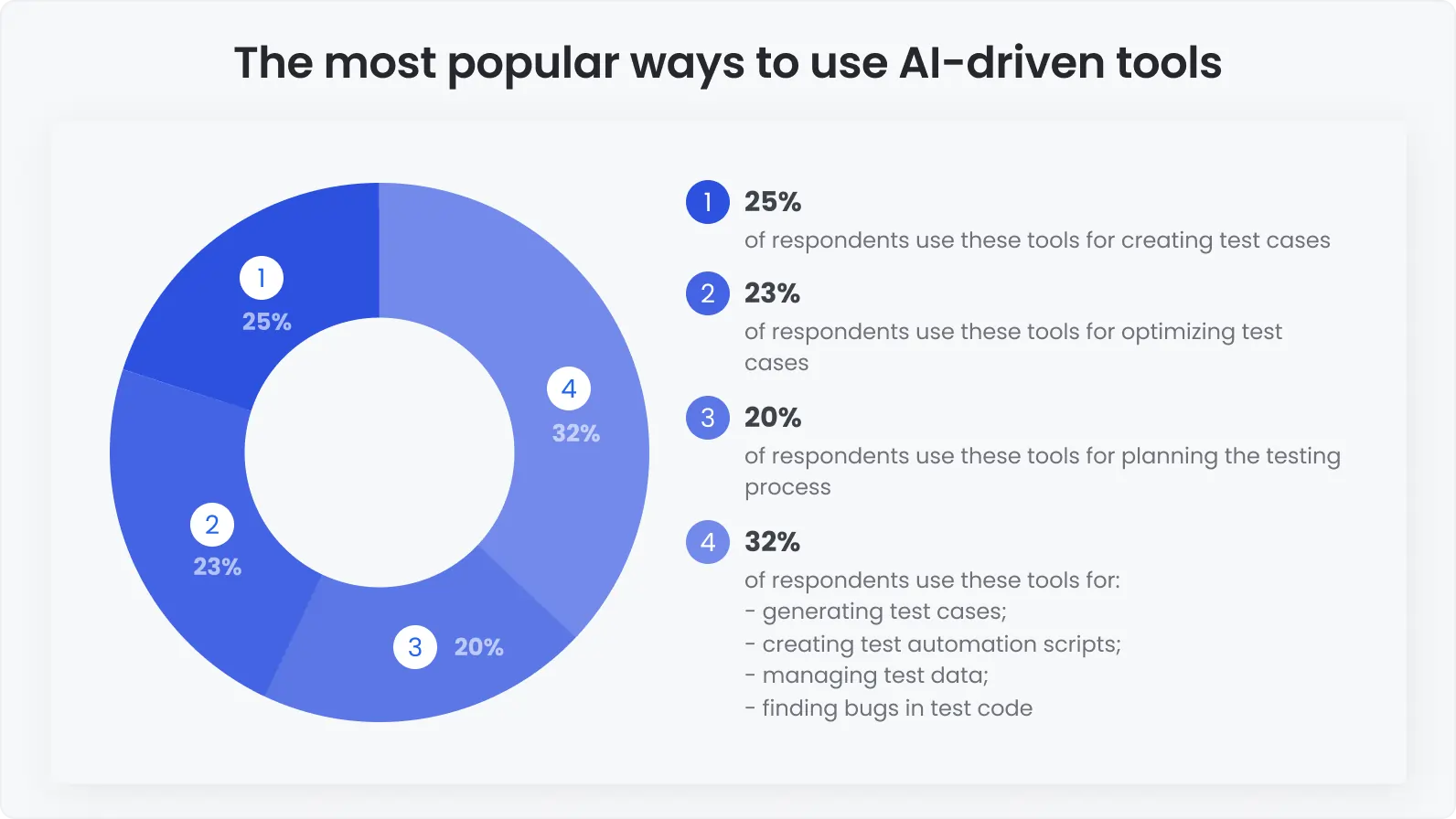
Creating test cases (25% of respondents use these tools for this purpose). Generative AI models allow the automation of this routine work. Thus, it is possible to accelerate the workflow and make the product test coverage as complete as possible with less time.
Optimizing test cases (23%). “Smart” tools can improve the quality of software testing. With their help, specialists can, for example, check if there are repeated test cases and delete them, remove or correct different test cases that check the same features or properties, analyze the code for “gaps” in coverage, and so on. All this helps to make QA process more high-quality and comprehensive.
Planning the testing process (20%). AI-driven tools can make good assistants in building a strategy. For example, predictive algorithms can identify problematic areas of code that need to be inspected primarily. Also, artificial intelligence can help prioritize test cases based on information about business goals, requirements for the application, code update history, previous test results, and other data.
According to another study, the following ways of using AI are the most popular among companies-respondents:
generating test cases;
creating test automation scripts;
managing test data;
finding bugs in test code.
Promising methods of using AI in QA
The above-described ways of using AI in testing software are not the only ones existing, but just the most popular ones. However, some methods are currently not so widespread but have a very high potential for further development. Here are a few examples of promising ways to use AI to improve the processes:
Self-healing test automation. According to a survey by TestRail, only 3% of surveyed companies are currently using this technique. However, this is a very promising method of using artificial intelligence in QA. It is an AI-based technology where automated test scripts adjust to UI and back-end updates in the product automatically to continue working efficiently despite constant updates.
UI testing. Using AI to test the visual side of applications has not yet become extremely popular. However, the tools for this already exist. They use ML and AI algorithms to analyze user behavior and, based on that, identify UI flaws and suggest ways to fix them.
Integrating AI in CI/CD pipelines for ongoing testing. When working under agile methodologies, continuous integration, delivery, and deployment are applied. It involves maximum automation of processes to accelerate and optimize them. Introducing AI into this pipeline can help a lot by enabling adaptive test case prioritization based on real-time code updates, autonomous test execution, and automated performance monitoring.
Future trends and challenges of using AI for software testing
The introduction of AI-based tools into QA looks promising. Many companies are already actively and successfully using “smart” assistants to accelerate, optimize, and improve workflows. AI models are continuously evolving. Therefore, their use may become more and more effective and productive over time.
Next in this article, you can find out more about:
what future trends in the development of AI for software testing await us soon;
what challenges the introduction of “smart” tools in QA may be associated with.
The future of cutting-edge AI solutions that assist in QA
The AI adoption rate is expected to grow by 36.6% each year by 2030. As for software quality assurance, the following ways of using smart algorithms may get more popularity in the future:
Computer vision technologies usage to control the quality of UI/UX by making sure there are no incompatibilities, cumulative layout shifts, or other shortcomings.
Automatic defect detection using advanced AI-powered technologies like deep learning-based static code analysis (SAST) and runtime anomaly detection.
Autonomous testing with minimal human intervention driven by AI technologies like reinforcement learning, deep learning models, and self-healing test automation scripts.
Challenges of implementing AI in testing
While the AI-powered approach helps to improve the quality assurance process, it is still not perfect. It can also be associated with certain difficulties and risks. Here are some of them:
Complexities associated with the introduction of new technologies. The right selection, implementation, and usage of effective tools may require having AI expertise. It may also be necessary to allocate some initial budget to put AI-powered tools to work.
Cultural resistance. Members of the QA team or key stakeholders may have a conservative approach to workflows. Because of this, it can be difficult to convince them of the necessity and benefits of using AI-based tools.
Employee adaptation and training. For team members to get used to and learn how to use “smart” assistants properly, you need to allocate some time and budget to adapt and train them.
Bias and quality issues. Some AI-based models may have problems with bias or inadequate results due to being trained on insufficient or low-quality information. This can lead to inaccurate defect predictions or test coverage gaps.
Safety issues. Smart tools usage in process automation often involves sharing sensitive information. Therefore, there is a risk of its leakage.
To avoid these difficulties and risks, it is essential to choose the right, high-quality, and safe, AI tools. It may also be necessary to order the services of external specialists with expertise in artificial intelligence to help implement this technology into your processes.
Prospects and reality: Balancing them for successful AI integration
Though the latest AI software testing news looks very promising and attractive, it is important to find the balance between expectations and reality. The introduction of AI into testing processes is very propitious. However, at this stage of technology development, it is not a panacea for all workflow problems and certainly not an option for replacing human employees. It is vital to understand that to get good results, it is necessary to:
Choose good, high-quality, safe, and trustworthy AI tools.
Properly set them to work correctly on your project.
Provide human supervision to eliminate possible problems and inaccuracies.
Factors to consider when introducing AI in QA
AI-based assistants can bring benefits only with the right choice and implementation. While incorporating new technologies into your software testing processes, it is better to take into account the following points:
The quality of the AI tools you choose. Favor those options that have all the needed functionality and strong security measures. Also, preferably use solutions that were trained on high-quality information. This ensures that the tool is accurate and bias-free.
Compatibility with tools you already use. You will most likely need to integrate new tools with those already in active use by your QA team. So make sure in advance that it can be done without any problems.
Objectives and profitability. Determine right away why you are going to implement new tools and whether it will be beneficial to your business.
QA team adaptation. Introduce new technologies gradually and adapt your testing professionals to the changes. Take care of continuous staff development and training. This will help avoid cultural resistance and ensure that specialists know how to properly use the full potential of AI tools.
To sum up
Artificial intelligence is transforming all work processes, including software quality assurance. Numerous companies are already actively using AI-based solutions to plan QA strategy and to create and optimize test cases. There are many more promising methods of using AI in quality assurance, such as self-healing test automation and computer vision UI testing.
Of course, “smart” algorithms cannot replace human specialists, but they can make excellent assistants for them. DeviQA team keeps pace with AI technology advancements and regularly implements new software QA methodologies. Contact us if you have any questions or need expert advice.
Team up with an award-winning software QA and testing company
Trusted by 300+ clients worldwide